Unveiling the Fluidity of Liquid Neural Networks
by
-
5 minutes read
-
March 30, 2021
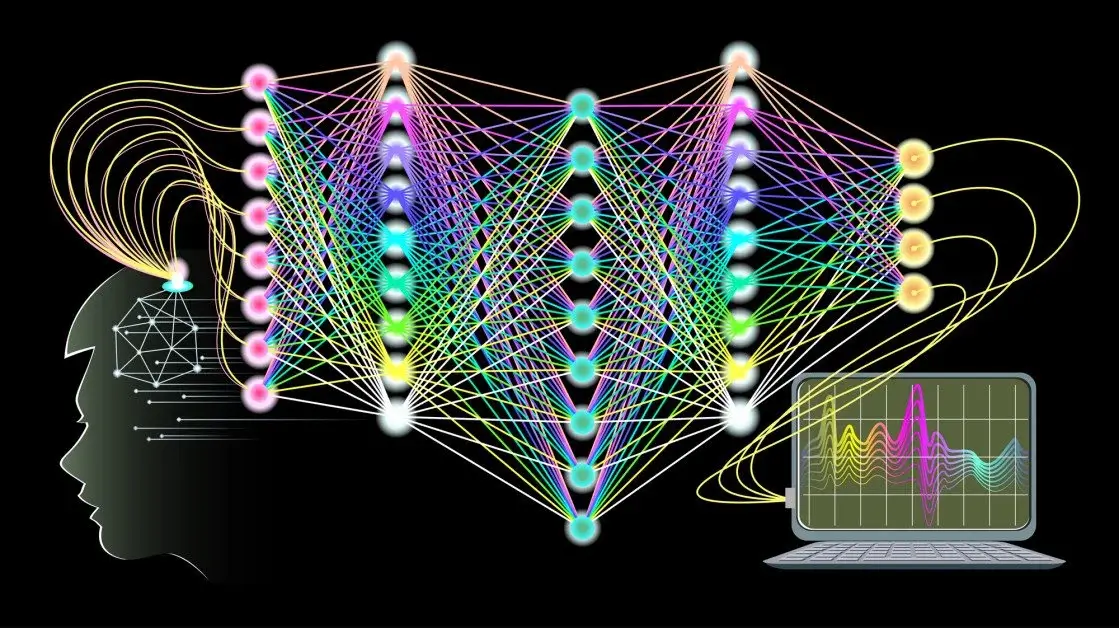
Introduction
In the realm of artificial intelligence, innovation is a constant, and new frontiers are continually being explored. One such avant-garde concept that has been making waves is the intriguing paradigm of Liquid Neural Networks (LNNs). Imagine a neural network that functions more like a dynamic, ever-changing entity than a static model—this is the essence of Liquid Neural Networks.
The Genesis of Liquid Neural Networks:
Liquid Neural Networks, often referred to as LNNs, are a novel approach to artificial neural networks that draw inspiration from the behavior of liquids. The conventional neural networks we are familiar with consist of layers of interconnected nodes, each layer transforming its inputs and passing them forward. In contrast, LNNs blur the lines between layers, introducing a continuous flow of information akin to the movement of fluids. This fluidic behavior leads to networks that can adapt, morph, and reconfigure themselves in response to different inputs, fostering an entirely new level of dynamism.
The Fluid Dynamics Analogy:
At the heart of Liquid Neural Networks lies their analogy to fluid dynamics. Much like how liquids adjust their shape and flow to fit the container they‘re in, LNNs adapt their structure and connections based on the data they‘re processing. Instead of rigid layers, LNNs incorporate a dynamic reservoir of interconnected processing units. These units interact in complex ways, enabling the network to exhibit behaviors such as temporal processing, sequence prediction, and pattern recognition with unprecedented flexibility.
Key Characteristics and Advantages:
- Adaptability:
LNNs thrive in scenarios where the data distribution changes over time. Their fluidic architecture allows them to adjust their internal configurations to match the characteristics of incoming data streams. This adaptability lends itself well to applications like online learning and real-time data analysis. - Temporal Processing:
One of the standout features of LNNs is their natural aptitude for processing temporal data. The continuous flow of information through the network makes them adept at tasks such as time series analysis, language modeling, and video processing. - Robustness:
The fluidic nature of LNNs imparts a level of robustness to noisy or incomplete inputs. The networkcs interconnectedness helps propagate useful information even when certain parts of the input data are corrupted. - Innovation and Collaboration:
Resource Efficiency: Traditional neural networks often demand significant computational resources during training and inference. LNNs, by virtue of their inherent adaptability, can achieve comparable performance with fewer parameters and computations. - Creativity and Innovation:
LNNs hold the potential to revolutionize the concept of creativity in AI systems. Their fluid structure can simulate associative thinking, potentially leading to breakthroughs in fields like art, music, and literature generation.
Challenges and the Road Ahead:
As with any groundbreaking concept, Liquid Neural Networks are not without challenges. Designing effective training algorithms for LNNs, ensuring stability in dynamic architectures, and understanding the optimal balance between adaptability and stability are areas that require further
As we venture further into the world of Liquid Neural Networks, we open doors to a new era of AI capabilities. The dynamic, fluidic nature of these networks brings us closer to achieving AI systems that can truly simulate the adaptability and complexity of the human brain. From real-time data analysis to creative endeavors, the potential applications of LNNs are as diverse as they are exciting.
As we venture further into the world of Liquid Neural Networks, we open doors to a new era of AI capabilities. The dynamic, fluidic nature of these networks brings us closer to achieving AI systems that can truly simulate the adaptability and complexity of the human brain. From real-time data analysis to creative endeavors, the potential applications of LNNs are as diverse as they are exciting.
Conclusion
In a landscape where AI is becoming increasingly integrated into our lives, Liquid Neural Networks offer a refreshing perspective—one that challenges traditional static neural network architectures and invites us to embrace the fluidity of thought and information. As research progresses and technologies evolve, we might witness the dawn of a new era in AI, where networks ebb and flow like digital currents, unraveling new avenues of innovation that we‘re only just beginning to fathom.