Leveraging AI for Credit Risk Management: Amplifying Profits and Mitigating Losses (Part 2)
by
-
5 minutes read
-
March 30, 2021
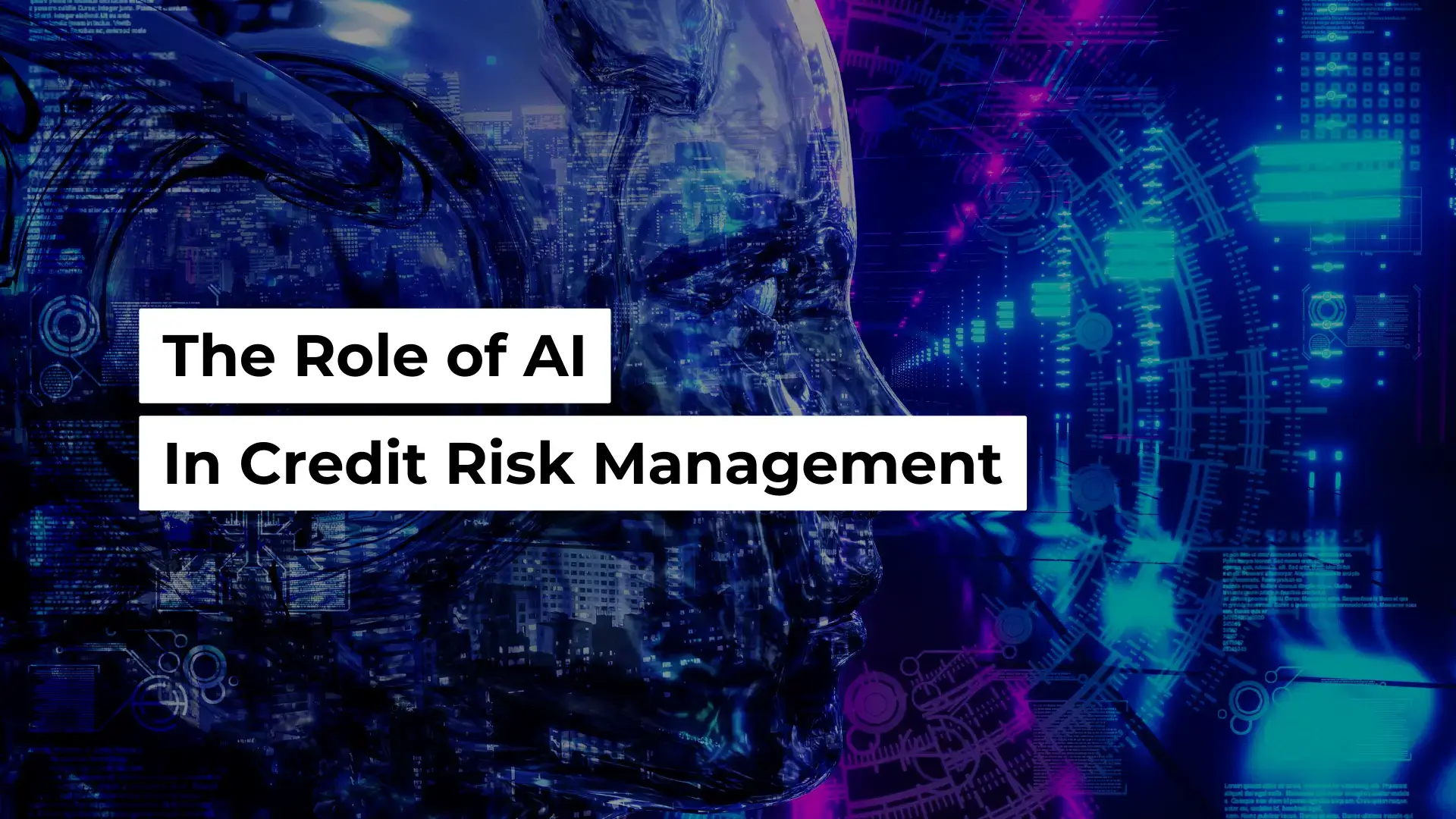
Challenges of Credit AI
1. Data Privacy and Security Concerns
Deploying artificial intelligence in credit risk management involves managing extensive amounts of confidential financial data, giving rise to apprehensions regarding potential data privacy issues and security breaches.
To safeguard the privacy of consumer information and maintain trust, it is crucial to enforce robust data protection protocols and adhere strictly to relevant data privacy regulations.
To safeguard the privacy of consumer information and maintain trust, it is crucial to enforce robust data protection protocols and adhere strictly to relevant data privacy regulations.
2. Regulatory Compliance Complexity
Successfully incorporating AI into credit risk management within the intricate regulatory framework of the United States presents a considerable challenge. Meeting evolving regulatory standards while harnessing AI technologies requires a thorough grasp of legal frameworks and continual adjustment to shifting compliance norms.
3. Algorithmic Bias and Fairness
The presence of algorithmic biases in AI models utilized for credit risk evaluation constitutes a notable challenge. Biased models have the potential to yield discriminatory results, jeopardizing the fairness of credit determinations.
Mitigating algorithmic biases involves rigorous testing, frequent audits, and the adoption of ethical AI practices. These measures are imperative to guarantee fair treatment for all customers.
Mitigating algorithmic biases involves rigorous testing, frequent audits, and the adoption of ethical AI practices. These measures are imperative to guarantee fair treatment for all customers.
4. Integration and Adaptation Costs
Incorporating AI technologies into current credit risk management systems frequently involves a significant initial investment and continuous operational expenses.
Financial and logistical challenges arise from the necessity for specialized expertise, infrastructure upgrades, and employee training. Addressing these challenges demands meticulous budgeting and strategic planning.
Financial and logistical challenges arise from the necessity for specialized expertise, infrastructure upgrades, and employee training. Addressing these challenges demands meticulous budgeting and strategic planning.
5. Technological Complexity and Maintenance
Due to their sophistication, AI systems require continuous maintenance, updates, and monitoring to ensure optimal accuracy and performance. Effectively managing technical complexities, addressing system failures, and staying informed about technological advancements are crucial to prevent disruptions in credit risk management processes and maintain a competitive edge in the dynamic financial landscape.
6. Customer Acceptance and Trust
The integration of AI in credit risk management can elicit customer concerns regarding transparency, accountability, and the dependability of automated decision-making processes.
Establishing customer trust involves transparent communication, providing clear disclosure of AI-driven processes, and showcasing the advantages of AI in enhancing credit risk assessment. These efforts are essential for fostering customer acceptance and loyalty.
Establishing customer trust involves transparent communication, providing clear disclosure of AI-driven processes, and showcasing the advantages of AI in enhancing credit risk assessment. These efforts are essential for fostering customer acceptance and loyalty.
7. Ethical and Legal Implications
Addressing the ethical and legal ramifications of AI-driven credit risk management involves considerations such as data usage, customer consent, and the ethical implications of automated decision-making. A thorough understanding of ethical frameworks and adherence to established legal guidelines are imperative to guarantee the responsible and compliant use of AI technologies.
Effectively navigating these challenges is essential for multinational firms seeking to employ AI in credit risk management in the US. This approach ensures sustainable growth, regulatory compliance, customer trust, and long-term success in the continuously evolving financial landscape.
Effectively navigating these challenges is essential for multinational firms seeking to employ AI in credit risk management in the US. This approach ensures sustainable growth, regulatory compliance, customer trust, and long-term success in the continuously evolving financial landscape.
Overcoming Challenges of Credit AI
1. Data Privacy and Security Concerns
- Establish robust data encryption and implement stringent access controls to protect sensitive financial information effectively.
- Regularly conduct comprehensive security audits and invest in advanced cybersecurity measures to proactively prevent data breaches.
- Sustain consumer confidence and meet regulatory standards by adhering to data privacy laws
2. Algorithmic Bias and Fairness
- Formulate a specialized team to monitor regulatory changes and ensure the prompt adaptation of AI systems to comply with evolving standards.
- Cultivate partnerships with legal experts and regulatory authorities to gain insights into regulatory expectations, ensuring alignment with compliance requirements.
- Commit to substantial investment in comprehensive training programs to educate employees on the latest regulatory developments and compliance protocols.
3. Algorithmic Bias and Fairness
- Utilize diverse data sets and integrate bias detection algorithms to identify and rectify potential biases within AI models.
- Promote diversity and inclusivity within AI development teams to encourage a holistic perspective, thereby mitigating the risk of biased algorithmic outcomes.
- Establish transparent and explainable AI models that empower stakeholders to comprehend the rationale behind credit decisions, fostering trust in the system‘s fairness.
4. Integration and Adaptation Costs
- Conduct a thorough cost-benefit analysis to assess the potential long-term advantages of incorporating AI into credit risk management.
- Allocate resources to invest in scalable AI infrastructure and prioritize technologies that seamlessly integrate with existing systems to minimize disruption and additional costs.
- Provide comprehensive training courses to enhance the skills of staff, ensuring a smooth transition to AI-powered procedures.
5. Technological Complexity and Maintenance Costs
- Create a robust maintenance schedule and invest in continuous monitoring tools to promptly identify and address technical issues.
- Stay updated on the latest developments and best practices in AI maintenance and management by fostering alliances with AI technology suppliers and consultants.
- To effectively embrace emerging AI solutions and adapt to technological changes, encourage a culture of continuous learning and innovation within the organization.
6. Customer Acceptance and Trust
- Give priority to transparent communication with customers about the incorporation of AI in credit risk management, emphasizing the benefits of AI- driven processes in ensuring fair and accurate credit assessments.
- Provide educational resources and personalized support to address customer concerns, building trust in the reliability and integrity of AI-powered credit risk management.
- To effectively meet customer expectations and preferences, customize AI-driven services by implementing feedback mechanisms to gather customer insights and preferences.
7. Ethical and Legal Implications
- Create an ethical framework for the deployment of AI that aligns with established industry standards and legal guidelines.
- Conduct regular ethical audits and reviews to verify compliance with ethical principles and legal requirements governing AI applications in credit risk management.
- Cultivate a culture of responsible AI usage, prioritizing ethical decision-making processes to uphold the integrity and trustworthiness of AI-driven credit risk management practices
Through the implementation of these strategies, multinational firms can adeptly navigate the challenges linked to AI adoption in credit risk management. This approach fosters a secure, ethical, and technologically advanced credit ecosystem, promoting sustainable growth and instilling customer trust.
Use Cases of credit AI
1. Automated Credit Scoring and Underwriting
By analyzing a wealth of consumer data, encompassing payment history, credit utilization, and other relevant financial indicators, artificial intelligence (AI) facilitates automated credit scoring and underwriting processes.
This capability leads to more precise and streamlined credit assessments, empowering your firm to make well-informed decisions on loan approvals and interest rates while simultaneously mitigating the risk of default.
This capability leads to more precise and streamlined credit assessments, empowering your firm to make well-informed decisions on loan approvals and interest rates while simultaneously mitigating the risk of default.
2. Fraud Detection and Prevention
The integration of AI in credit risk management enables real-time detection and prevention of fraudulent activities by analyzing transaction patterns and customer behavior.
The implementation of AI-driven fraud detection systems plays a pivotal role in safeguarding your firm and customers from potential financial losses, thereby fostering a secure and trustworthy financial ecosystem.
The implementation of AI-driven fraud detection systems plays a pivotal role in safeguarding your firm and customers from potential financial losses, thereby fostering a secure and trustworthy financial ecosystem.
3. Personalized Customer Experiences
AI-powered tools play a key role in crafting personalized customer experiences through the delivery of tailored financial solutions, customization of credit offerings, and responsive customer support.
By strategically incorporating AI in customer interactions, your firm can elevate customer satisfaction, foster long-term relationships, and improve overall customer retention rates.
By strategically incorporating AI in customer interactions, your firm can elevate customer satisfaction, foster long-term relationships, and improve overall customer retention rates.
4. Predictive Analysis for Risk Management
The incorporation of predictive analytics powered by AI empowers your firm to proactively assess and manage credit risks. Artificial intelligence (AI) can predict future market fluctuations and customer behaviors by analyzing historical data and market trends.
This capability enables your business to make informed, data-driven decisions that effectively minimize potential financial risks and maximize profitability.
This capability enables your business to make informed, data-driven decisions that effectively minimize potential financial risks and maximize profitability.
5. Portfolio Management and Optimization
AI plays a pivotal role in portfolio management and optimization by offering insights into the performance of credit portfolios and identifying high-risk segments.
Through the utilization of AI-driven tools, your firm can make well-informed decisions regarding portfolio diversification, restructuring, and risk mitigation strategies, ensuring a more balanced and profitable credit portfolio.
Through the utilization of AI-driven tools, your firm can make well-informed decisions regarding portfolio diversification, restructuring, and risk mitigation strategies, ensuring a more balanced and profitable credit portfolio.
6. Regulatory Compliance and Reporting
AI simplifies the process of regulatory compliance and reporting by automating compliance checks, ensuring accurate, and timely reporting. Through the utilization of AI-driven systems, your firm can streamline regulatory compliance processes, decrease the risk of penalties, and ensure adherence to complex regulatory requirements.
7. Market Trend Analysis and Forecasting
AI empowers comprehensive market trend analysis and forecasting by processing substantial volumes of market data and macroeconomic indicators.
Utilizing AI-powered analytics, your firm can acquire valuable insights into market dynamics, anticipate future trends, and make strategic business decisions. This ensures a competitive edge and sustainable growth in the dynamic financial landscape.
By exploring these AI use cases in credit risk management, multinational firms can harness the power of AI technology to streamline processes, enhance decision-making capabilities, and foster a more secure and profitable credit environment.
Utilizing AI-powered analytics, your firm can acquire valuable insights into market dynamics, anticipate future trends, and make strategic business decisions. This ensures a competitive edge and sustainable growth in the dynamic financial landscape.
By exploring these AI use cases in credit risk management, multinational firms can harness the power of AI technology to streamline processes, enhance decision-making capabilities, and foster a more secure and profitable credit environment.